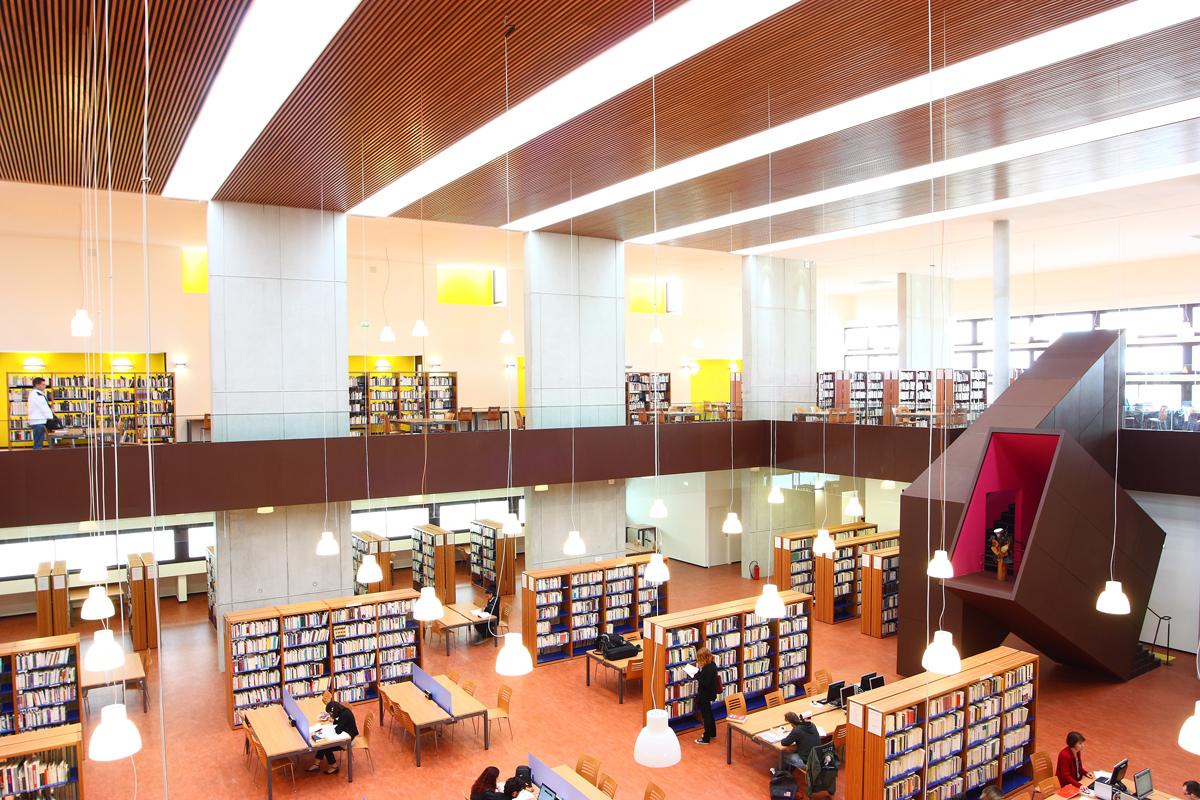
La présentation sera assurée par Anass El Moadine:
Poisoning-Attack Detection for Deep Learning Models
Modern Deep Learning DL models can be trained in various ways, including incremental learning. The idea is that a user whose model has been trained on his own data will perform better on new data. The model owner can share its model with other users, who can then train it on their data and return it to the model owner. However, these users can perform poisoning attacks PA by modifying the model’s behavior in the attacker’s favor. In the context of incremental learning, we are interested in detecting a DL model for image classification that has undergone a poisoning attack. To perform such attacks, an attacker can, for example, modify the labels of some training data, which is then used to fine-tune the model in such a way that the attacked model will incorrectly classify images similar to the attacked images, while maintaining good classification performance on other images. As a countermeasure, we propose a poisoned model detector that is capable of detecting various types of PA attacks. We demonstrate the performance of our method on a variety of architectures and in the context of a DL model for mass cancer detection in mammography images.