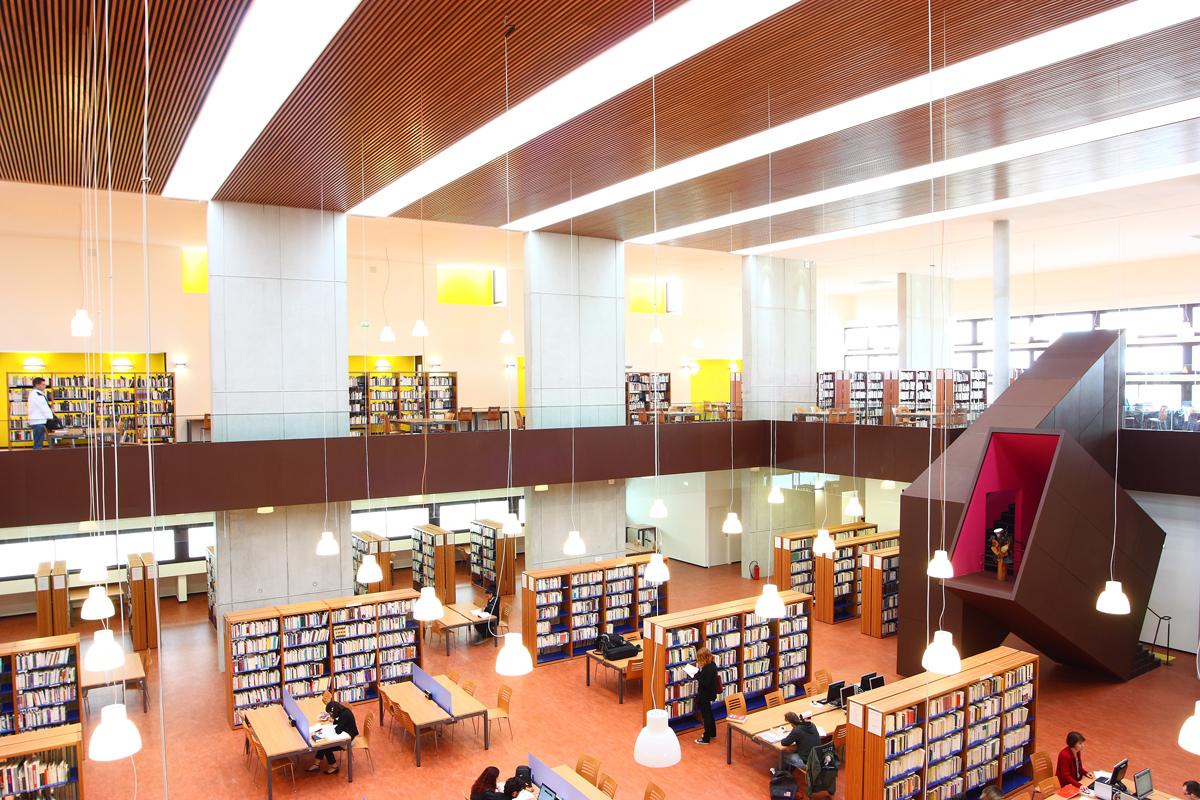
Le séminaire mensuel sera présenté par Arnaud Boutillon.
Segmentation of the pediatric musculoskeletal system serves as an essential pre-processing step to guide clinical decisions, as the generated 3D models of muscles and bones help clinicians to evaluate pathology progression and optimally plan therapeutic interventions. However, the accuracy and generalization performance of automatic segmentation models trained on individual domains are limited due to the restricted amount of annotated pediatric data. To address this issue, we developed and optimized a segmentation model on multiple datasets, arising from different parts of the anatomy, in a multi-task and multi-domain learning framework. This approach allows to overcome the inherent scarcity of pediatric data while benefiting from a more robust shared representation. The proposed segmentation network comprises shared convolutional filters, domain-specific batch normalization parameters that compute the respective dataset statistics and a domain-specific segmentation layer. Furthermore, a supervised contrastive regularization is integrated to further improve generalization capabilities, by promoting intra-domain similarity and forcing inter-domain margins in latent space. We evaluated our contributions on two sparse, unpaired (from different patient cohorts), and heterogeneous pediatric musculoskeletal datasets of the ankle and shoulder joints. We obtained promising results for the task of delineating pediatric bones from magnetic resonance (MR) images.